Here CodeAvail statistics experts will explain to you about what is the use of regression in statistics with examples in detail.
Regression in Statistics
Table of Contents
In Statistics, Regression is a set of statistical procedures for assessing the connections between a reliant variable (frequently called the ‘result variable’). And at least one independent factor (regularly called ‘indicators’, ‘covariates’, or ‘features’). Also, the most widely recognized type of regression analysis is linear regression.
In which a specialist finds the line that most closely fits the information as per a particular scientific rule. For example, the technique for common least-squares processes the special line (or hyperplane). However, That limits the whole of squared divisions between the genuine information and that line (or hyperplane).
For explicit mathematical reasons, this permits the analyst to calculate the conditional desire (or population normal estimation) of the dependent variable. When the free factors take on a given set of qualities.
There are several types of regression in statistics, but before moving to the details of them. Let’s get some information on what is a statistical regression.? Also, regression is the branch of statistics that plays an essential role in predicting analytical data.
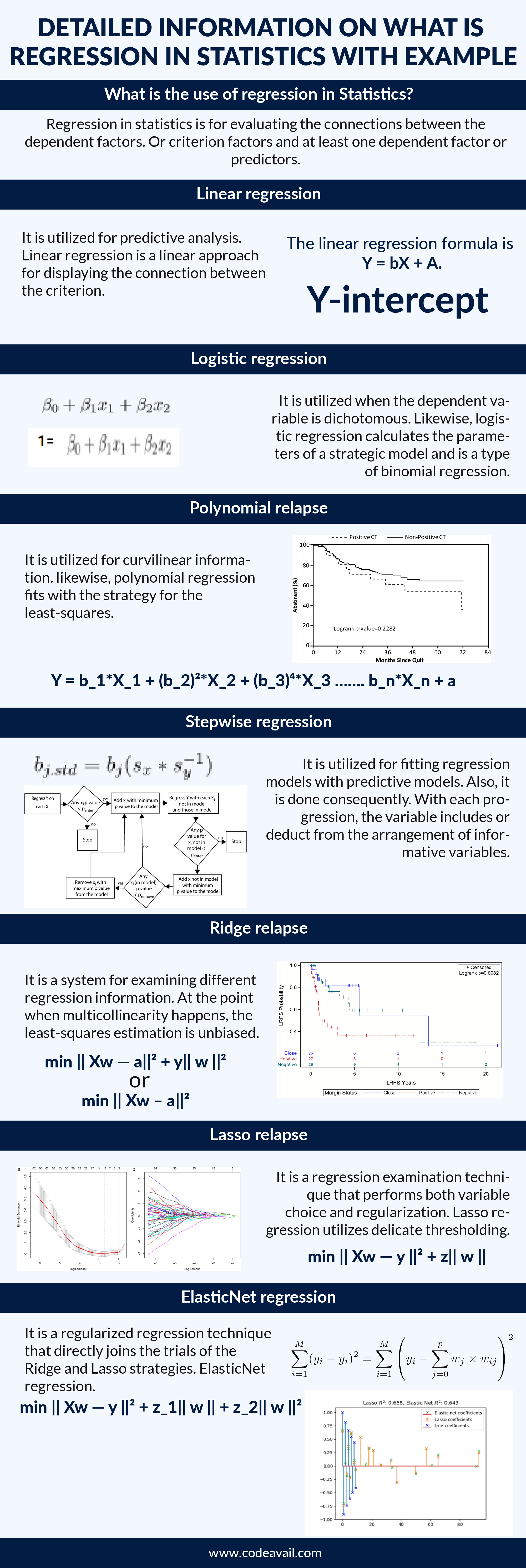
What is the use of regression in Statistics?
Regression in statistics is for evaluating the connections between the dependent factors. Or criterion factors and at least one dependent factor or predictors. Regression clarifies the changes in rules corresponding to changes in select predictors. The conditional desire for the models dependent on indicators. Where the normal value of the dependent factors is given when the independent factors are changed. Likewise, three significant uses for regression are deciding the quality of indicators, measuring an impact, and trend forecasting.
Regression Types :
- Linear regression
- Polynomial regression
- Logistic regression
- Stepwise regression
- Ridge regression
- ElasticNet regression
- Lasso regression
Linear regression:
It is utilized for predictive analysis. Linear regression is a linear approach for displaying the connection between the criterion. Or the scalar response and the various explanatory or predictors variables. Linear regression concentrates on the conditional probability distribution of the response provided the predictors’ values. For linear regression, there is a risk of overfitting.
The linear regression formula is Y = bX + A.
Where b= slope of the line, b = Y-intercept
Logistic regression:
It is utilized when the dependent variable is dichotomous. Likewise, logistic regression calculates the parameters of a strategic model and is a type of binomial regression. Calculated/logistic regression is utilized to manage information. That has two potential models and the connection between the standards and the indicators. For example, the condition for Logistic regression is:
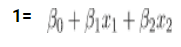
Polynomial relapse:
It is utilized for curvilinear information. likewise, polynomial regression fits with the strategy for the least-squares. The objective of the analysis of regression to show the normal estimate of a dependent variable y with respect to the independent variable x. However, the condition for polynomial regression is:
Y = b_1*X_1 + (b_2)²*X_2 + (b_3)⁴*X_3 ……. b_n*X_n + a
Stepwise regression:
It is utilized for fitting regression models with predictive models. Also, it is done consequently. With each progression, the variable includes or deduct from the arrangement of informative variables. The methods for stepwise regression are forward choices, in reverse exclusion, and bidirectional exclusion. For example, the stepwise regression formula is:
Ridge relapse:
It is a system for examining different regression information. At the point when multicollinearity happens, the least-squares estimation is unbiased. And a level of inclination is added to the regression estimation, and an outcome, ridge regression decreases the standard mistakes. For example, the equation for ridge regression is:
min || Xw — a||² + y|| w ||²
OR
min || Xw – a||²
Where X determines the feature variables, w represents the weights, and a represents the ground truth.
A bias matrix technique utilizes to sum the least square equations. And finally, the addition of squares can reduce and perform the value of low variance parameters. The bias matrix is also necessary for scalar multiple identical matrices where the optimum value requires to get selected.
Lasso relapse:
It is a regression examination technique that performs both variable choice and regularization. Lasso regression utilizes delicate thresholding. Also, regression chooses just a subset of the given covariates to use in the last model. Lasso regression is
min || Xw — y ||² + z|| w ||
This method helps to utilize it for feature collections where the variable or set and parameters are chosen for sample constructions. It takes the appropriate zeroes and features with inappropriate values that are applied to avoid overfitting. And also makes it possible to learn faster. It is both a regularization sample and a feature selection.
ElasticNet regression:
It is a regularized regression technique that directly joins the trials of the Ridge and Lasso strategies. ElasticNet regression is utilized to help portfolio, metric learning, and vector machine improvement. The trial work is given by:
min || Xw — y ||² + z_1|| w || + z_2|| w ||²
How is Regression Analysis Used in Forecasting
The regression technique for forecasting includes analyzing the connection between two unique factors. Also known as dependent and free factors. Assume that you need to forecast future deals for your firm and you’ve seen that business rise or fall.
Depending upon whether the GDP goes up or down. (The GDP is the aggregate of all things considered and benefits delivered inside a country’s borders. In the U.S., it is determined quarterly by the Commerce Department.)
Your business, at that point, would be the dependent variable. Since they are depending upon the GDP, which is the free variable. (A free factor is a variable against which you are estimating something by correlation – your deals for this situation.) You would need to make sense of how intently these two factors – sales and GDP – are connected. If the GDP goes up 2 percent, what amount does your business rise?
Example of regression Analysis
In spite of the fact that this sounds complicated, it’s quite basic. You could essentially look back at the movement of the GDP in the last quarter or over the most recent three-month time span. And compare it with your marketing figure.
In all actuality, the government detailed that the GDP developed 2.6 percent in the final quarter of 2018. If your business rises 5.2 percent during that similar period. You’d have a really smart thought that your sales by and large ascent at double the pace of GDP development because:
5.2 %(your deals)/2.6%= 2
The “2” implies that your sales are increasing at double the pace of the GDP. You should return two or three additional quarters to be certain this pattern proceeds, state for a whole year. Assume you sell vehicle parts, forklifts, or wheat.
It would be the same despite the product or services you sell. Since you realize that your sales are expanding at double the pace of GDP development. At that point, if the GDP builds 4 percent the following quarter, your sales will probably rise 8 percent. On the off chance that the GDP goes up 3 percent, your deals would almost rise 6 percent and so on.
Along these, regression analysis can be a significant device for forecasting sales and assist you with deciding. If you have to build supplies, work, production hours, and any number of different factors.
Conclusion
This blog we have provided all the information regarding what is the use of Regression in statistics with examples. We have also mentioned 7 types of regression that involve linear, ridge, lasso, and much more. All these are used to analyze the several variable sets in case of multicollinearity and dimensionality.
As a result, If you still find any difficulty related to your statistic assignments then you can contact our customer support executive anytime and from anywhere in the world. We have a team of specialists who can give you high-quality data within the slotted time.
Our services are available for 24*7 at an affordable price that helps you to score good grades in your academics. Also, if you want do my statistics assignment help, you can asks our experts.